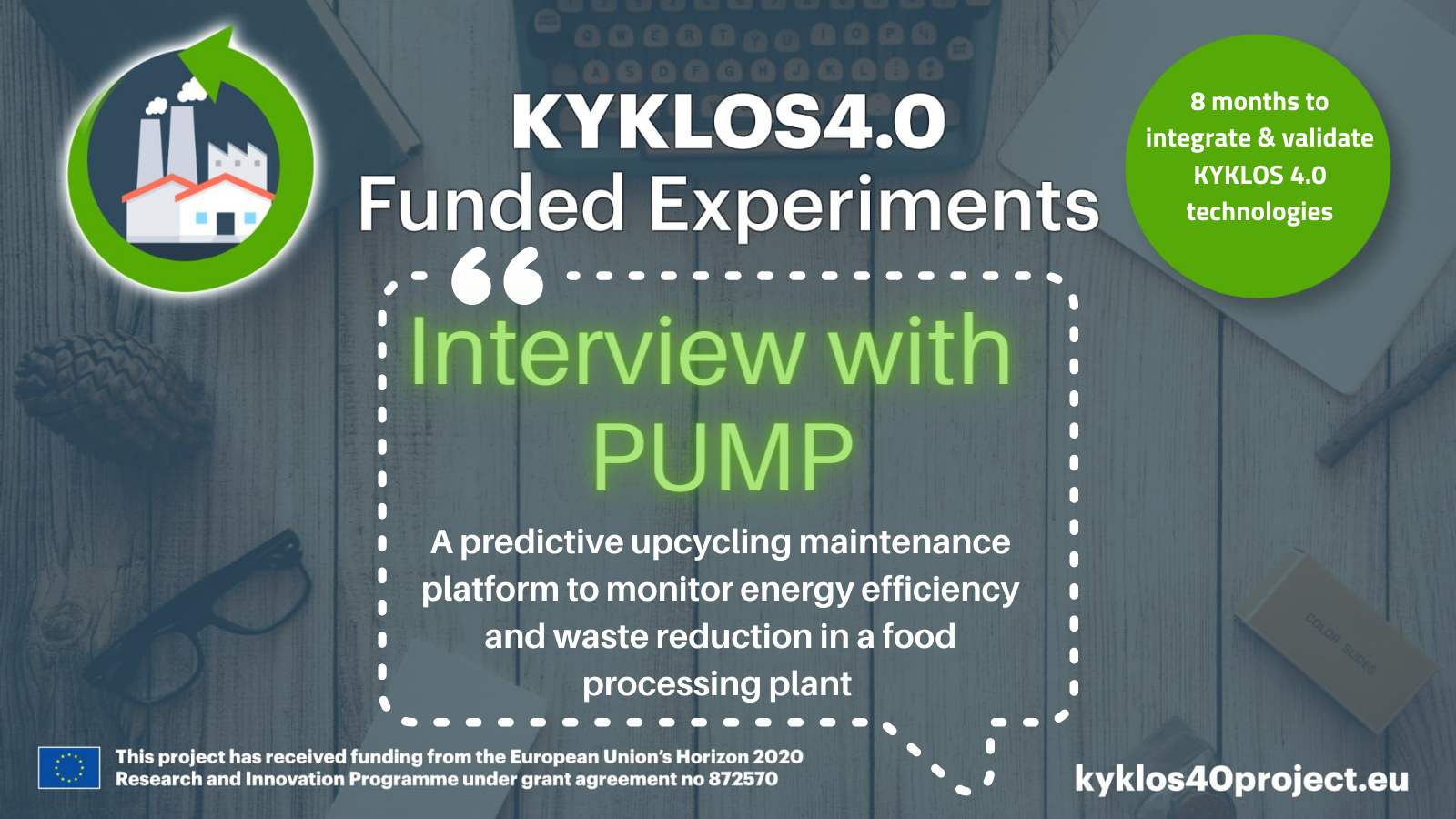
26 Jul Interview with the PUMP experiment
The KYKLOS4.0 Open Call #2 is funding seventeen experiments that are developing technological solutions of value for the manufacturing domain.
The project will be releasing a series of interviews over the course of the coming weeks with the objective of promoting the work being implemented in these experiments and allowing our readers to understand potential uptake of the solutions being developed by them.
This is the thirteenth of the seventeen interviews with the experiments funded under the open call. Today, we interview the POET4POEM experiment.
* * * * *
Explain your project in one sentence.
PUMP aims to apply energy monitoring tools and advanced Machine Learning models to analyse the patterns in electrical loads of machinery equipment, disaggregate component loads and detect deviations from normal operations in a food processing plant, aiming for energy efficiency and maintenance planning optimisation, enhancing its circularity approach.
How is KYKLOS4.0 project and the selected services (and components) bringing value to your project?
PUMP focuses on predictive maintenance and energy efficiency and will harness the holistic approach of KYKLOS 4.0 to offer enhanced features through the collaborative platform. Data collected from the factory shop-floor regarding mainly the energy consumption of the equipment and the production process, will be used alongside the technical components of the industrial partners of the KYKLOS 4.0 platform. The PUMP technical partners will leverage their developed technologies such as energy disaggregation and energy analytics to support energy efficiency and efficient machinery health monitoring (MhM). PUMP will utilise various KYKLOS 4.0 technical components, combine them with the already developed tools and technologies of the PUMP partners to maximise the efficiency and enhance the overall solution. More specifically, the LCA simulations engine component (KYKLOS TC4.4.1) will support process efficiency and reduction of waste by modelling the production process and getting insightful analytics related to the environmental impact of each recipe of the food processing plant, exploring the alternatives towards optimisation; the Maintenance Scheduler Component (KYKLOS TC3.6.1) will be utilised towards assisting scheduled maintenance planning actions and creating alerts for the technicians and the Decision Support System (KYKLOS TC8.1.1) will enable the seamless integration of the various components outputs to derive insights towards targeted actions and calculation of circular indicators.
How is your solution contributing to circular manufacturing?
Circular economy is a core concept for the PUMP experiment, as it aims at highlighting the repairability and recyclability of Electrical Motor Driven Systems (EMDs), which will prolong their life, reduce equipment waste and contribute to the food processing factory’s circular economy plan, resulting to a more sustainable use of resources, without causing any reliability or safety issues. The system will utilise machine learning to analyse the different discrepancies in a motor’s energy consumption pattern to prevent unexpected breakdowns. By preventing unexpected breakdowns there is a quantity of product and raw materials that is saved and will be used instead of becoming waste. Additionally, the overall predictive and proactive scope of the experiment can decrease the overall waste of EMDS systems, their hazardous wastes such as oil, magnets etc. and prolong their end of life.
More specifically, the PUMP experiment will target various circular economy KPIs, aiming (i) to reduce valuable materials and energy losses, by enhancing the upcycling percentage of motor based equipment and its reusability rate; and (ii) reduce waste and emissions levels from the production process utilising the LCA tool and the modelling of the process that the pilot partner will try to optimise. The solution aims to assist industrial plants to receive Energy Efficiency tips, optimise the production process, and enable more efficient and targeted maintenance planning.
How will your solution be replicated and used in other manufacturing environments?
The PUMP solution can be replicated and used in other manufacturing environments by following the same principles and steps taken in the food processing plant. The first step would be to identify the key equipment and machinery that (i) consumes the most energy, (ii) often needs maintenance, and (iii) identifying the process line that has the highest potential for optimisation.
The energy consumption patterns of the machinery can be monitored using advanced energy monitoring tools, which can be implemented in any manufacturing environment. Once the energy consumption patterns have been analysed, advanced machine learning models will disaggregate component loads and detect deviations from normal operation in order to prevent unexpected breakdowns and optimise maintenance planning, which can save both time and resources. Additionally, the use of predictive and proactive maintenance can decrease the overall waste of the machinery and hazardous wastes.
The Circular Economy concept can be used to reduce valuable materials and energy losses, enhance the upcycling percentage of machinery and its reusability rate, prolonging their life, while at the same time reduce emissions levels from the production process. Life Cycle Assessment (LCA) tools can be used to model the manufacturing process and optimise it for energy efficiency and waste reduction. Additionally, the industrial plants will receive energy efficiency tips, enabling more efficient and targeted maintenance planning.
What advice would you give to companies wishing to make their manufacturing processes more circular?
The first step for a company that wants to make its manufacturing process more circular is to analyse their entire production process, starting from the raw materials up to the final product, in order to identify areas where circularity indicators can be improved. This will help to identify opportunities for reuse, recycling, or upcycling, and to determine where waste is being generated and can be minimised.
The next step would be the prioritisation of waste and emissions reduction throughout their production process, by implementing more efficient processes, reducing packaging waste, and using sustainable materials. Moreover, end-of-life of products and materials should be taken into consideration through design for disassembly, using recyclable materials, and developing products that can be easily repaired or refurbished.
Utilisation of state-of-the-art technologies such as machine learning and data analytics combined with Life-Cycle-Assessment can be used to simulate and then optimise processes and reduce waste. This can include using predictive maintenance to extend the life of equipment, optimising production processes to reduce waste, and use data to track material flows and identify opportunities for circularity.
* * * * *
About the PUMP project
The PUMP project – Predictive Upcycling Maintenance Platform – is implemented by three partners: VRUMP Industrial IoT Solutions (Greece), PLEGMA LABS S.A. (Greece), HELIOS BAKERY (P&E TAKOUDIS – PSKAFIDAS O.E.) (Greece)
Summary: The main objective of the project is to apply non-intrusive energy tracking tools and ML models to analyse patterns in electrical loads of machinery equipment, disaggregate component loads and detect deviations from normal operation in a food processing plant