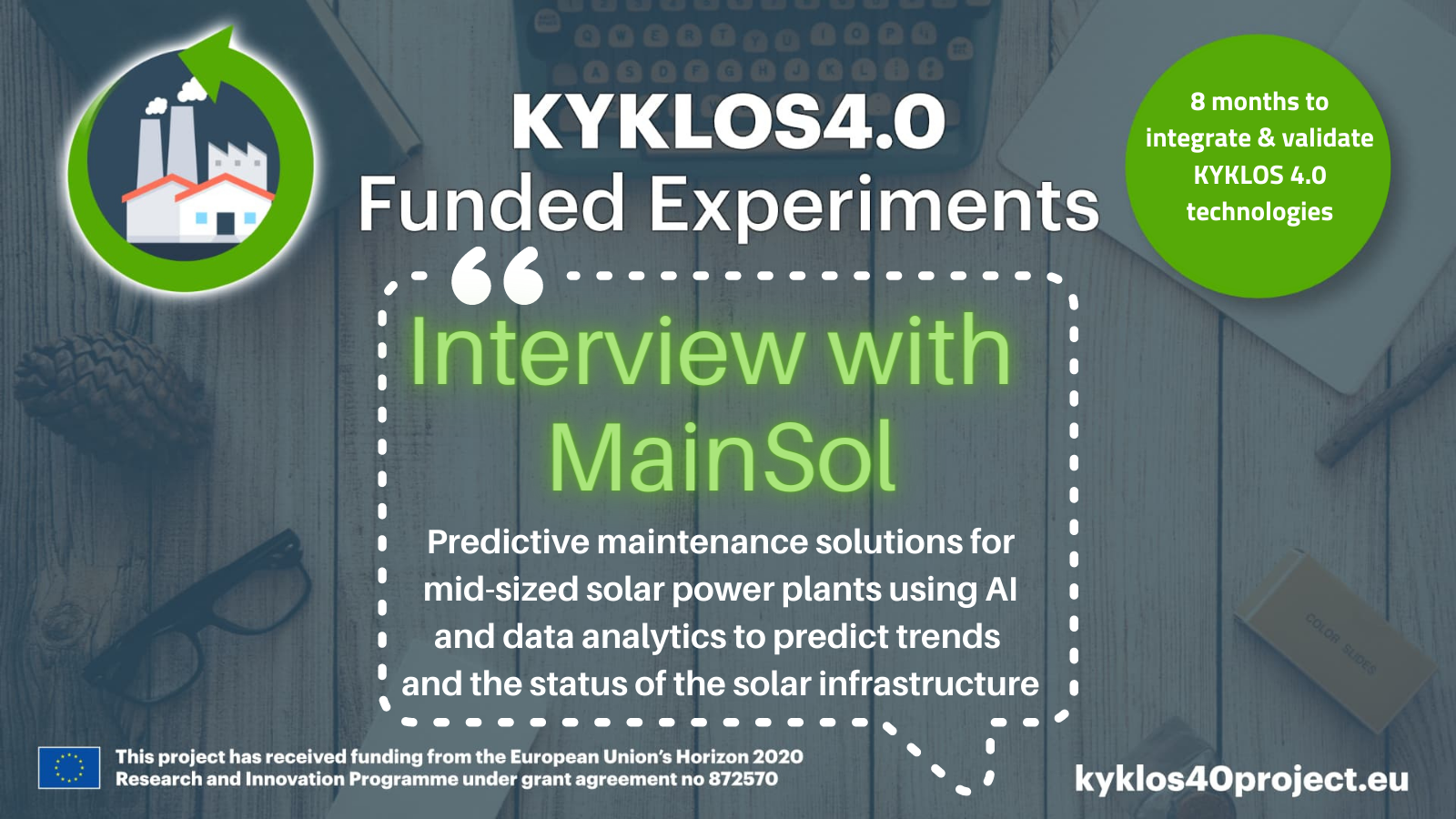
22 Jun Interview with the MainSol experiment
The KYKLOS4.0 Open Call #2 is funding seventeen experiments that are developing technological solutions of value for the manufacturing domain.
The project will be releasing a series of interviews over the course of the coming weeks with the objective of promoting the work being implemented in these experiments and allowing our readers to understand potential uptake of the solutions being developed by them.
This is the eleventh of the seventeen interviews with the experiments funded under the open call. Today, we interview the MainSol experiment.
* * * * *
Explain your project in one sentence.
MainSol’s vision is to create a new generation of the predictive maintenance solutions for middle-sized solar power plants which use the advanced AI and data analytics methods for predicting/analysing not only the trends in generated solar energy, but also the status of the entire solar and manufacturing infrastructure enabling a holistic predictive maintenance.
How is KYKLOS4.0 project and the selected services (and components) bringing value to your project?
The solution adopts KYKLOS 4.0 services and components from the category Product Optimisation, in particular Predictive Maintenance service in order to enable advanced data analytics for industry 4.0, leading to more informed decisions. The LCA component will be used for enabling LCA-based calculation over the real-time data. The goal is to extend existing AI-based services on streaming data (namely, fast thinking – a service for detecting unusual behavior) with the product life cycle context. MainSol will contribute to the objective of validation in real world scenarios the KYKLOS 4.0’s value proposition: manufacturing-driven services and digital components to increase manufacturing circularity and production efficiency.
How is your solution contributing to circular manufacturing?
The main goal of the usage of environmental sensors is to understand the impact of the energy consumption on the environment (going towards eco-friendly quality). Therefore environmental sensing is important for calculating correlation models between: operational mode, energy consumption and environmental impact. This will enable us to provide/quantify the benefits of the system in the context of decreasing a) Energy consumption and b) Environmental impact. All is put in the context of the maintenance of solar power plants, since a more efficient usage of the solar panels will be not only improving the usage of renewable energy sources, but rather improving the environmental aspects.
How will your solution be replicated and used in other manufacturing environments?
Potential impact is huge, since planned results have the potential to contribute to a radical shift in the quality management process, transformed into a smart and environmentally friendly process for optimal decisions built on the trade-offs borne in quality-related and sustainability-related KPIs. Through the pilot in the food domain, this proposal will extend the list of use cases/scenarios offered by KYKLOS 4.0 and test data can be offered to other partners. Moreover, the planned solution will enhance data analytics services in the KYKLOS 4.0 Platform with an AI-based innovative service for early detection of change points / instabilities in the process, which offers new opportunities for solving challenging industry problems.
What advice would you give to companies wishing to make their manufacturing processes more circular?
Basic advice would be the following:
- Start collecting real time data (production, energy, environment-related) in a systematic way.
* * * * *
About the MainSol project
The Mainsol project – Holistic predictive maintenance of solar power systems for sustainable manufacturing – is implemented by two partners: PHOENIX (Serbia), DESIGN (Serbia)
Summary: The main objective of the project is to create a new generation of predictive maintenance solutions for mid-sized solar power plants that use advanced artificial intelligence and data analytics methods to predict/analyse not only trends in solar energy generated, but also the state of the entire solar and manufacturing infrastructure, enabling holistic predictive maintenance