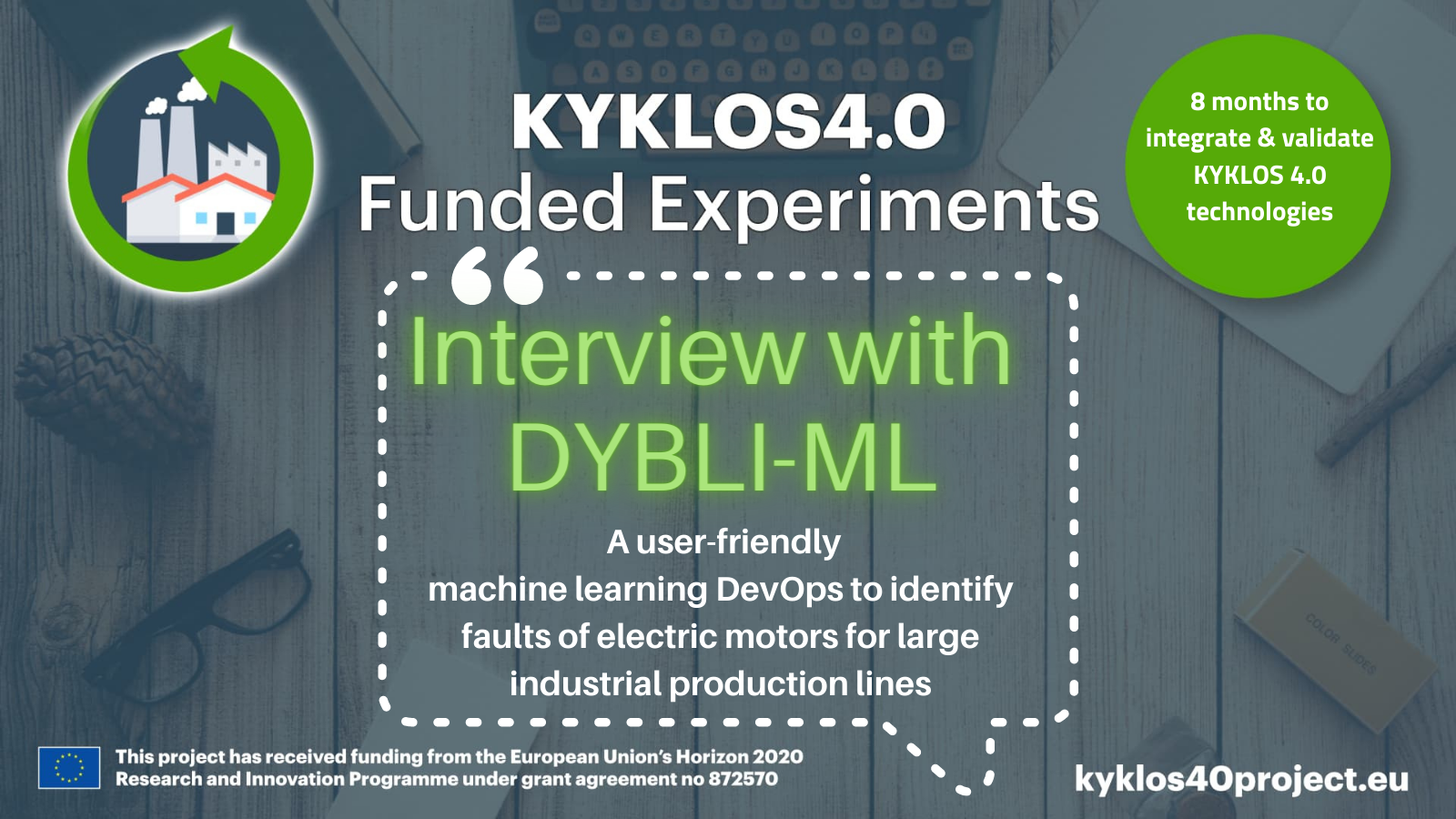
12 May Interview with the DYBLI-ML experiment
The KYKLOS4.0 Open Call #2 is funding seventeen experiments that are developing technological solutions of value for the manufacturing domain.
The project will be releasing a series of interviews over the course of the coming weeks with the objective of promoting the work being implemented in these experiments and allowing our readers to understand potential uptake of the solutions being developed by them.
This is the seventh of the seventeen interviews with the experiments funded under the open call. Today, we interview the DYBLI-ML experiment.
* * * * *
Explain your project in one sentence.
DYBLI-ML provides a user-friendly MLOps solution for fault detection in electric motors for industrial production lines, with a long-term vision of a device-agnostic ML dashboard to predict failures. The project aims to build a community ecosystem for exchanging trained ML models, reducing redundant work and improving accuracy.
How is KYKLOS4.0 project and the selected services (and components) bringing value to your project?
KYKLOS 4.0 promotes the digitisation of the manufacturing industry, which aligns with our vision of bringing MLOps to the industrial sector. The scalability of the novel circular manufacturing ecosystem that KYKLOS 4.0 proposes also complements our long-term goal of creating an ecosystem around our MLOps solution that is device agnostic and can scale to other industries. Additionally, KYKLOS 4.0 provides expertise ranging from IoT integration to MLOps, allowing us to collaborate and leverage each other’s strengths for a future-proof product that is already validated as a viable solution. KYKLOS 4.0 provides the ML models in TC 3.2.1 fault detection system and the Deep Learning Toolkit in TC 4.1.1, which can be added to our full-fledged IoT platform, Monidas. This will enhance our solution and allow for dynamic model storage and retraining pipeline. The maintenance scheduler TC 3.6.1 provided by KYKLOS will enable real-time support and validation of our ML models, ensuring that our solution is accurate and reliable. Overall, the collaboration with KYKLOS 4.0 and its selected services and components brings significant value to our project in terms of expertise, scalability, validation, and real-time support. We are excited to work with them and believe that our proposed solution will benefit the manufacturing industry.
How is your solution contributing to circular manufacturing?
Our solution, DYBLI-ML, contributes to circular manufacturing by promoting the reuse of machine learning models, which reduces waste and energy consumption. By providing a platform for industrial partners to share ML models, we enable knowledge transfer and increase the predictive accuracy of our models. Our system also allows for early detection of machine failures, which reduces energy losses and prevents expensive machine repairs or replacements. By catching issues before they cause significant damage, we also reduce the amount of material waste generated by discarded or replaced machines. In addition, our focus on fault detection for electric motors aligns with the circular economy principle of extending the lifespan of products. By detecting and repairing faults, our solution enables the continued use of existing machinery, reducing the need for new equipment and the resources required to manufacture it. Furthermore, our user-friendly ML dashboard allows technicians to monitor and predict failures, enabling proactive maintenance and further extending the lifespan of industrial equipment. Ultimately, our solution contributes to a more sustainable and circular approach to manufacturing by reducing waste, extending the lifespan of existing equipment, and promoting knowledge sharing and reuse.
How will your solution be replicated and used in other manufacturing environments?
Our solution is built to be easily replicated and used in other manufacturing environments. We focus on eliminating complexity and providing a user-friendly platform for fault detection of electric motors in large industrial production lines. Our long-term vision is to create a user-friendly ML dashboard to allow technicians to monitor and predict failures, which could be utilised in other manufacturing environments. Additionally, our proposed solution benefits from a community of industrial partners who can share their trained ML models, allowing for a circular exchange of models across different manufacturing sectors. This can significantly reduce the amount of time and energy needed for customers who would otherwise have to set up and train their own independent systems. Moreover, our solution can be extended to other industries as we introduce different models for different problems. We have expertise ranging from IoT integration to MLOps, and our platform, Monidas, can handle data storage and visualisation. Adding dynamic model storage and retraining pipeline will allow us to scale our solution to other manufacturing environments with minimal effort. In conclusion, we are confident that our solution can be replicated and used in various manufacturing environments with ease, providing a reliable and cost-effective solution for fault detection and other services.
What advice would you give to companies wishing to make their manufacturing processes more circular?
To companies wishing to make their manufacturing processes more circular, we advise considering the full lifecycle of their products and equipment. This includes not only production and use but also maintenance, repair, and disposal. By adopting circular manufacturing practices, companies can reduce waste, increase efficiency, and improve sustainability.
* * * * *
About the DYBLI-ML project
The DYBLI-ML project – MLOps for sustainable Industry 4.0 with Fault Detection Models – is implemented by two partners: Colomba Link GmbH (Switzerland), LABORATOIRES PICHOT (France).
Summary: The main objective of the project is to create a user-friendly machine learning DevOps to identify faults of electric motors with a view to enable technicians to monitor and predict failures.