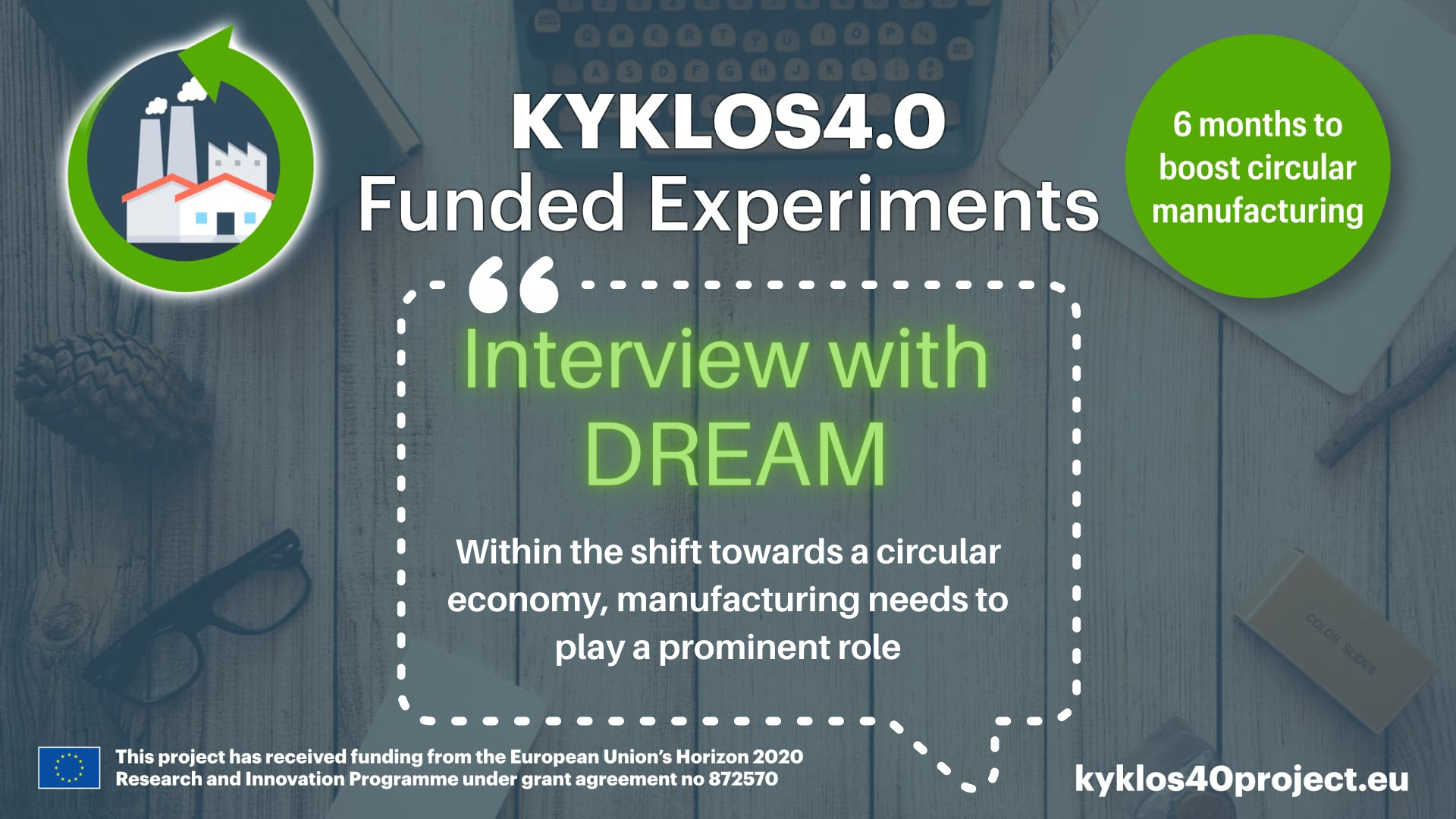
31 Mar Interview with the DREAM experiment
The KYKLOS4.0 Open Call #1 is funding seven experiments that are developing technological solutions of value for the manufacturing domain.
The project is releasing a series of interviews over the course of the coming weeks with the objective of promoting the work being implemented in these experiments and allowing our readers to understand potential uptake of the solutions being developed by them.
This is the fourth of seven interviews with the experiments funded under the open call. Today, we interview the DREAM experiment.
* * * * *
Explain your project in one sentence.
The DREAM project is developing a Hybrid data-driven (AI + physics-based) Decision Support Platform. This platform will help manufacturers in the assessment of the machine tool’s exploitable life for reuse, thus leading to circular manufacturing.
How is KYKLOS4.0 delivering value to your project?
KYKLOS4.0 is actively contributing to the extension of industrial networks while creating new contacts with European research centres. Thanks to the KYKLOS4.0 Production Equipment Clustering (PEC) component, DREAM was able to set up and perform unsupervised clustering with reference to a demo use-case. With respect to such an activity, synergies with other companies involved in the KYKLOS4.0 Open Call#1 have been established to enhance the effectiveness and scalability of the test/results.
How is your solution contributing to circular manufacturing?
Within the shift towards a circular economy, manufacturing needs to play a prominent role. As Machine Tools (MTs) play a relevant role in a wide range of manufactured products, the reuse of MTs is an essential strategy in this context. Containing operating cost (e.g. reducing machine tools downtime) and assuring quality are important aspects for the customers of machine tool builders and must be considered for an effective reuse of equipment. Quality, however, heavily depends on the condition of the equipment.
The reuse of machine tools is a practice that increased during the last decade. However, the assessment of the effective exploitable useful life at the end of the first lifetime (EO1L) is a tricky task, which is often not adequately performed. At EO1L of a machine tool, the assessment of its exploitable life for reuse is of paramount importance in a circular manufacturing approach.
The DREAM project will provide companies’ decision makers with a smart decision support platform to take the right decision at the right time, thus fostering a sustainable and resilient circular manufacturing approach.
How will your solution be replicated and used in other manufacturing environments?
Machine Tools technology is a critical element of manufacturing, as Machine Tools are at the starting point of almost every manufacturing activity. For this reason, the machine tool industry is a key enabling sector, which has a direct impact on the productivity and competitiveness of the European manufacturing sector and on the transition to a more circular economy. In addition to the machine tool industry, metalworking companies (especially users of milling machines) are a potential target for the application of the developed Hybrid data-driven (AI + physics-based) Decision Support platform. However, digitalisation and real-time diagnosis of machinery conditions make the solution easily replicable in a wider range of industrial applications, such as automated assembly lines, paper and plastic converting, the newspapers printing industry, the food and beverage industry.
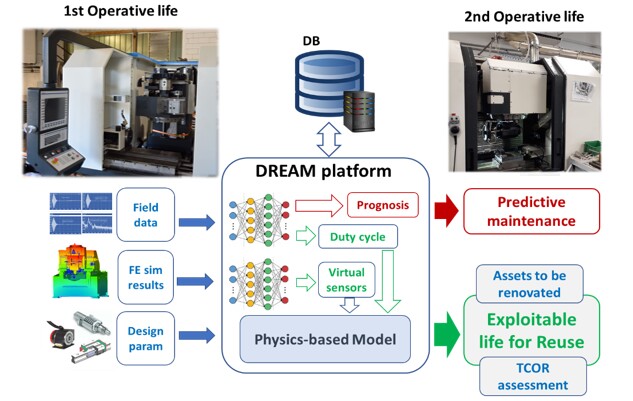
DREAM experiment scheme
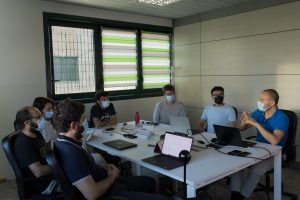
The ‘DREAM’ team
* * * * *
About the DREAM project
The DREAM project – Hybrid data-driven model for smart assessment of exploitable useful life of machine tools within circular manufacturing – is implemented by two partners from Italy: KITENERGY Srl. (Coordinator) and SCORTA Srl.
Summary: DREAM aims to develop a Hybrid data-driven (AI + physics based) Decision Support platform able to: (1) assess the actual exploitable life of machine components at EO1L; (2) identify component that need to be replace and or remanufactured; (3) assess the Total Cost of Reuse of machine tools (for the second lifetime); and (4) enable an effective predictive maintenance for the second life.