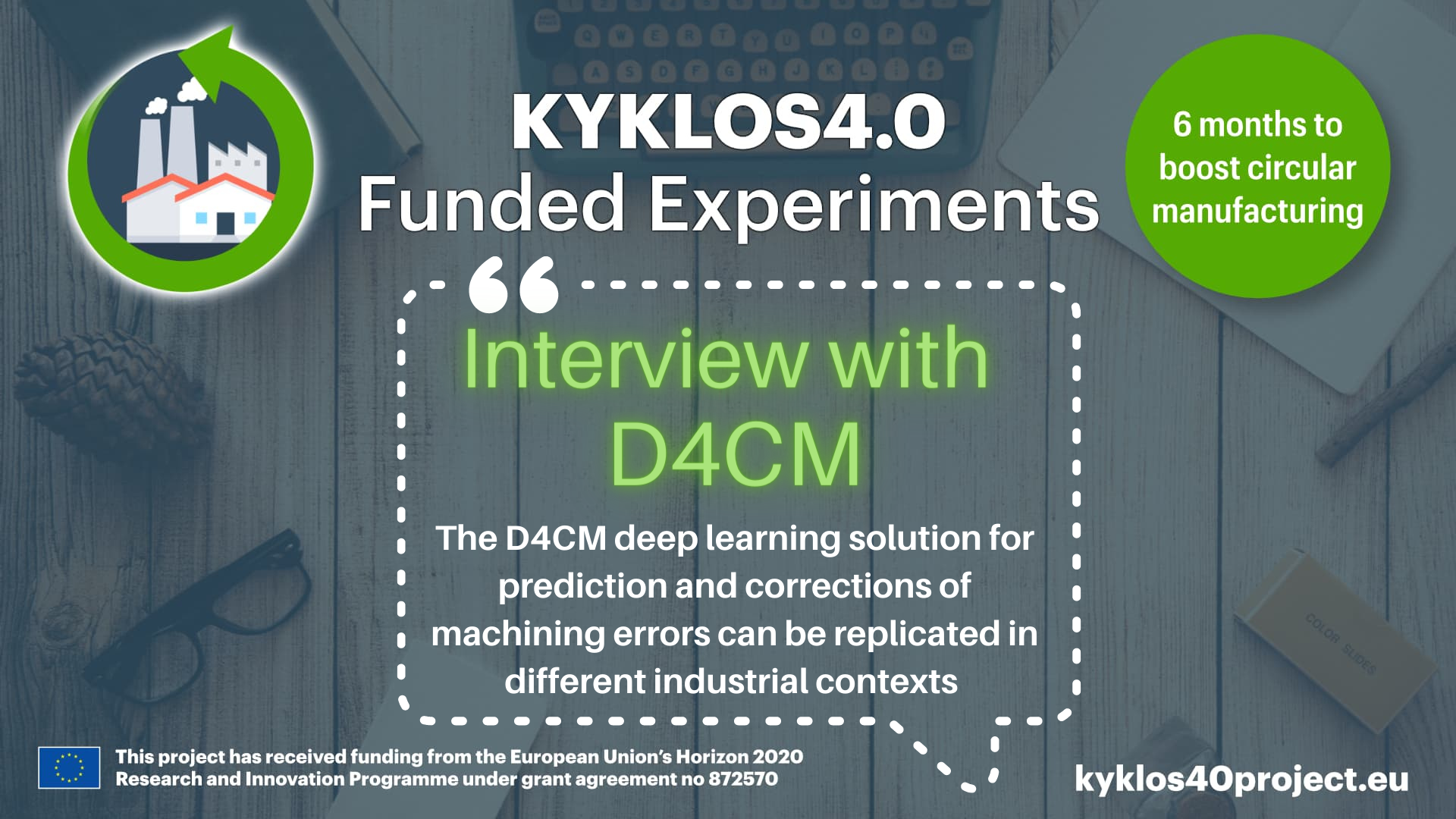
23 Mar Interview with the D4CM experiment
The KYKLOS4.0 Open Call #1 is funding seven experiments that are developing technological solutions of value for the manufacturing domain.
The project is releasing a series of interviews over the course of the coming weeks with the objective of promoting the work being implemented in these experiments and allowing our readers to understand potential uptake of the solutions being developed by them.
This is the third of seven interviews with the experiments funded under the open call. Today, we interview the D4CM experiment.
* * * * *
Explain your project in one sentence.
D4CM makes use of deep learning (DL) to increase the machining process quality and foster energy efficiency.
How is KYKLOS4.0 delivering value to your project?
The disruption of manufacturing caused by COVID-19 led to severe operational, social and financial consequences. Almost every sector has been affected, with generalised losses up to 30-50%. AI and CPS-based solutions are an essential part of re-fuelling rapid change and building resilience and sustainability in manufacturing. The D4CM experiment will provide manufacturers with solutions to respond more quickly, accurately, eco-friendly and successfully to COVID-19 disruptions.
In this context, the KYKLOS4.0 project is helping and supporting DC4M to bring R&D results and new services in a higher level network, enabling the quick transfer of generated skills and solutions across sectors, then fostering a rapid response of the overall EU economy.
How is your solution contributing to circular manufacturing?
The D4CM kit solutions (Sensors Fusion, DL Learner, DL Predictor, Adaptive Control) can eventually be exploited as part of a distributed CPS system, where tools, hardware and software can dynamically be added or removed, and dynamically exchange information and collaborate. At the end of the first life, when/if the machine is renovated/retrofitted for a second life, the ‘predictor’ module can be re-used as-is in the re-deployment stage, while the ‘Learner’ module will be re-trained to generate updated models/algorithms according to the renovation process. Such updated models are imported by the Predictor module (that runs on CNC) through the Predictive Model Markup Language (PMML) standard. Updated DL and control models can then both be re-used for 2nd life of machines and provide feedback to the Design stage for updating future machines designs (e.g. through the identification of critical thermal zones of the machines with respect to machining errors issues).
How will your solution be replicated and used in other manufacturing environments?
The D4CM deep learning solution for prediction and corrections of machining errors will be developed using a modular approach, comprising 2 modules. The “learner” module ingests huge datasets and trains the AI models/algorithms. The “predictor” module imports ML algorithms (through PMML standard) from the learner, and, based on real-time acquisition of field signals, provides the estimation of machining errors to be compensated by an adaptive control. Each module can be easily connected to a company database (both SQL and NoSQL), where relevant signals are stored. In particular, users can configure such modules to include/exclude features to be parsed, and include/exclude AI models to be trained. This modular and hierarchical approach will enable the proper scalability of the concepts according to user needs, and will then be replicated in different industrial contexts to foster circular manufacturing.
What advice would you give to companies wishing to make their manufacturing processes more circular?
- Have clear goals for circular manufacturing within the company: assess through a life cycle cost analysis the actual benefits that can be achieved via this approach (which could be much more than an enhancement of the brand image by being greener).
- Carefully select the proper enabling technology to reach the goals.
- In case of adoption of AI-based technologies, be sure to have (or to grow) the adequate skills inside the company, in order to properly manage their implementation.
- Be patient: economic return related to the adoption/implementation of circular manufacturing strategies could not be immediate: look at the medium and long-term perspective!
* * * * *
About the D4CM project
The D4CM project – Deep learning-based errors Detection to boos Data-Driven Circular Manufacturing – is implemented by two partners from Italy: Ce.S.I. Centro Studi Industriali (Coordinator) and PAMA Spa.
Summary: D4CM thus aims to develop, implement and validate a robust and confident CPS platform that includes an AI-based machine Errors Detection and Adaptive compensation models relying on Deep Learning techniques. These models are developed with an holistic (from Design stage to Renovation) and modular approach that enables a quick and effective retraining and re-deployment in case of reuse of equipment, to foster circular manufacturing. This is fully in line with KYLOS4.0 approach and addresses the Deep Learning subdomain.